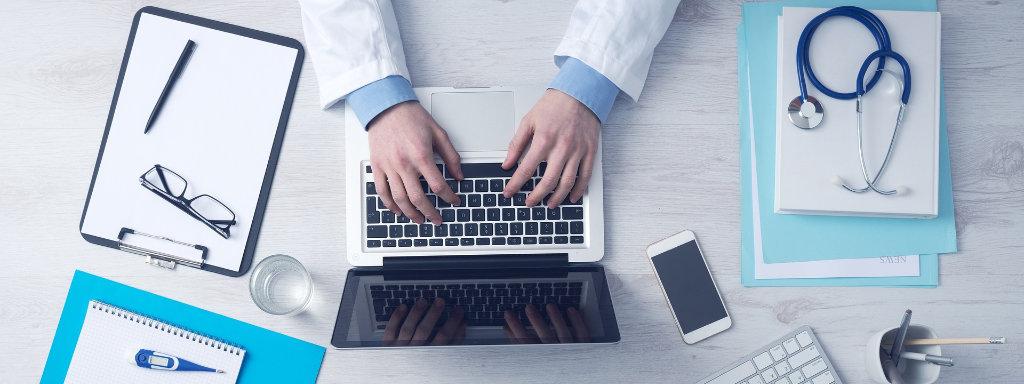
Submitted by S.A. Norwood on Mon, 14/04/2025 - 15:35
Synthetic data holds great promise, but considerable uncertainties remain about how to fully and responsibly realise its potential. Urgent collaboration between medical, ethical, and machine learning communities, and the public, is essential to develop standardised governance frameworks, appropriate-use guidelines, and consensus-based definitions of quality, safety, and fairness. The time to act is now.”Governing synthetic data in medical research: the time is now1
Cambridge researchers Dr Daniela Boraschi, Prof Mihaela van der Schaar, Dr Alessia Costa and Dr Richard Milne urge policy-makers to take action. Their recent article 'Governing synthetic data in medical research: the time is now', published in The Lancet Digital Health, asks scientists and regulators alike to consider how synthetic data should be used.1 But what is synthetic data? When, why and how is it currently used? And what considerations should we be taking into account in future?
What is synthetic data?
Synthetic data is information generated by artificial intelligence algorithms that mimics real-world data. It is based on existing data and aims to reproduce the characteristics and structure of the original data. Good quality synthetic data allows researchers to expand small datasets and should produce similar results to original data when analysed statistically.
Why use synthetic data in biomedical research?
Synthetic data is increasingly of interest in biomedical research for its capacity to provide extensive, anonymous datasets. These datasets are essential for developing and studying machine learning algorithms and AI applications, with several project-specific and publicly available datasets accessible for researchers to download.2,3,4
How is synthetic data currently used?
Synthetic medical data has applications in a number of areas and could address some problems posed by traditional data collection. Potential uses of synthetic medical data are currently being developed and explored by researchers, but could include:
Anonymous datasets to train AISynthetic datasets on cancer,5 cardiovascular diseases,6 and COVID-197 have been created using patient information, to help researchers train machine learning and AI models on data that does not contain any sensitive personal information. |
|
Replacing missing dataReal-world datasets often contain ‘censored’ data where a full set of data is not available if, for example, a patient pulls out of a study. Synthetic data can be used to ‘fill in’ missing data and allow for better statistical analyses.8 |
|
Expanding small datasetsSmall datasets can hinder statistical analysis and cause biases in AI. This is particularly a problem in healthcare data where information on underrepresented and minority groups is lacking. Synthetic data can be used to expand small datasets, allowing AI models to perform better for a wider range of people.9 |
How should we use synthetic data in the future?
Synthetic data has the potential to improve the openness, fairness, and efficiency of healthcare data analysis. However, as Dr Boraschi and her colleagues highlight in their recent paper1 and blog,10 there are a number of considerations that should be explored as the use of synthetic data becomes more common in biomedical research.
|
Data QualityHow can we measure the quality of a synthetic dataset and understand how well it reflects real populations and healthcare scenarios? |
PrivacyHow do we ensure that privacy is maintained and generative models do not reproduce any sensitive data?11 |
|
EquityHow do we apply findings based on synthetic data to patients? How do we test whether a model based on synthetic data performs better for one group of people than another? |
|
Transparency and AccountabilityHow should synthetic data be used compared with real-world data? How do we keep track of real vs synthetic data and ensure transparency? How do we establish accountability? |
In their paper the researchers call for action to start regulating synthetic data and ensure that these aspects are taken into account. Standardised frameworks for measuring the quality of synthetic data should be adopted, and guidelines for appropriate use should be produced. With these tools in hand, synthetic data will enable open, efficient, and equitable research, and the development of new AI-based technologies and practices.
References & Links
- Governing synthetic data in medical research: the time is now. The Lancet Digital Health (2025)
- Synthetic data in medical research. BMJ Medicine (2022)
- Synthetic data in health care: A narrative review. PLOS Digital Health (2023)
- Synthetic data generation methods in healthcare: A review on open-source tools and methods. Computational and Structural Biotechnology Journal (2024)
- Simulacrum v2 synthetic dataset. Simulacrum (2023)
- CPRD cardiovascular disease synthetic dataset. CPRD (2020)
- CPRD COVID-19 symptoms and risk factors synthetic dataset. CPRD (2021)
- SurvivalGAN: Generating Time-to-Event Data for Survival Analysis. Proceedings of Machine Learning Research (2023)
- Can You Rely on Your Model Evaluation? Improving Model Evaluation with Synthetic Test Data. NeurIPS Proceedings (2023)
- Synthetic Data and Healthcare: Ethics Q&A. Kavli Centre for Ethics, Science, and the Public (2025
- Are synthetic health data ‘personal data’. The PHG Foundation (2024)
Author Information
Credits & Acknowledgements
Many thanks to the authors Dr Daniela Boraschi, Prof Mihaela van der Schaar, Dr Alessia Costa and Dr Richard Milne and to Dr Fergus Imrie for sharing their thoughts and reviewing this article.